A comparison of seismic facies classification methods
By Brian Russell
GeoSoftware
brian.russell-contractor@cgg.com
Dr. Brian Russell
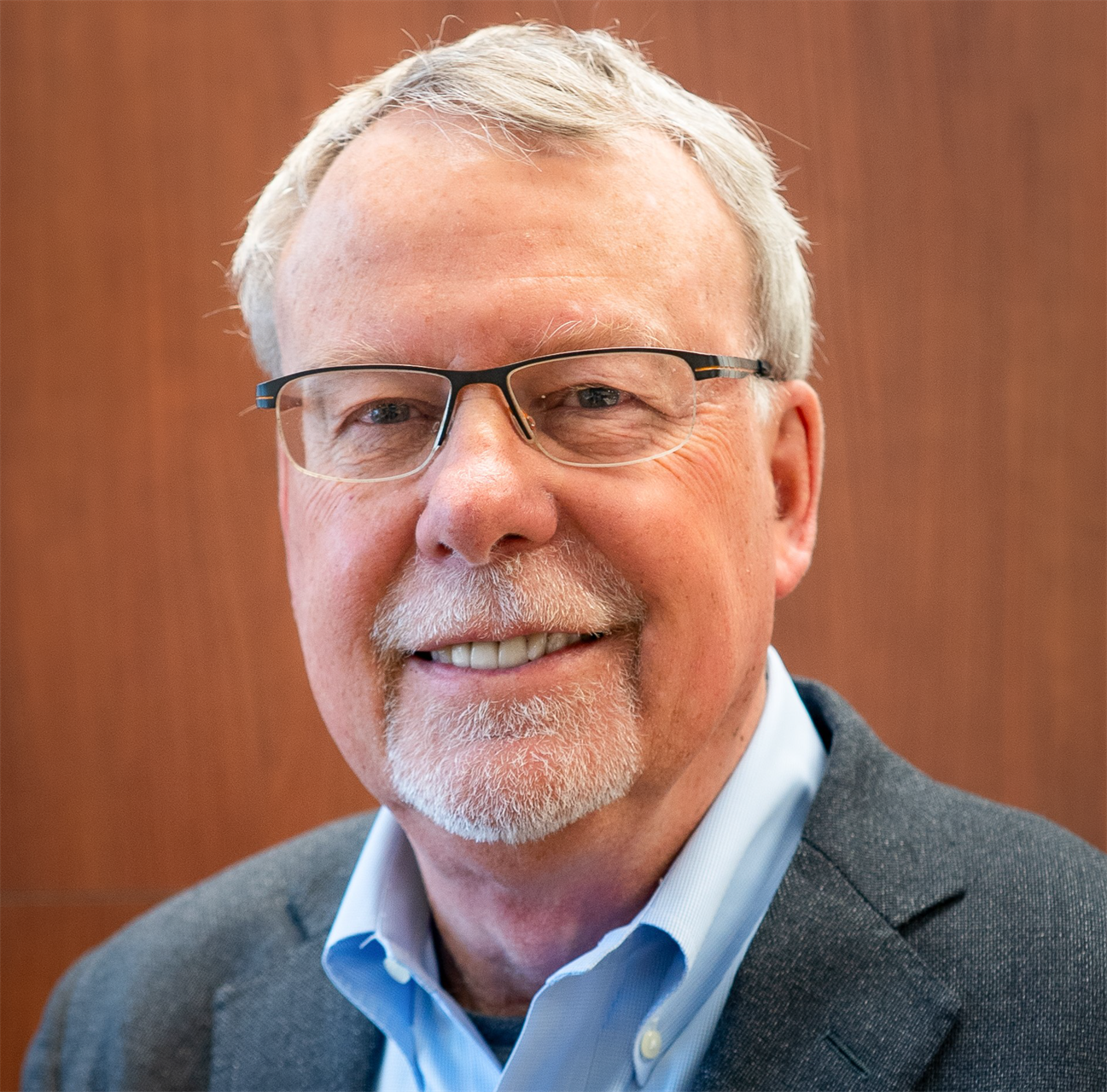
BIO
Brian Russell holds a B.Sc. from the University of Saskatchewan, a M.Sc. from Durham University in the UK, and a Ph.D. from the University of Calgary, all in geophysics. He joined Chevron Canada as an exploration geophysicist in 1975 and subsequently worked for Teknica Resources and Veritas Seismic before co-founding Hampson-Russell Software with Dan Hampson in 1987. HampsonRussell is now a subsidiary of GeoSoftware, where Brian is Vice President.
Brian is involved in research into AVO, rock physics, inversion and machine learning techniques as well as giving presentations throughout the world, currently via on-line platforms! He is a Past-President of both the SEG and Canadian SEG and has received Honorary Membership from both societies, as well as the Cecil Green Enterprise Award from SEG (jointly with Dan Hampson) and the 1999 CSEG Medal. Brian is also an Adjunct Professor in the Department of Geoscience at the University of Calgary and is registered as a Professional Geophysicist in the Province of Alberta.
ABSTRACT
In this study, I compare parametric and nonparametric statistical methods for seismic facies classification. First, I use a straightforward example that uses 2 seismic attributes, normalized Vp/Vs ratio and P-impedance, 2 facies and 10 data points. This problem includes several outliers that illustrate the advantages and disadvantages of each method. I then finish with a facies classification example from the Permian Basin.
For the 10-point example, I first apply least-squares classification, and k-Nearest Neighbor, or kNN, classification. The least-squares approach did a poor job of separating the classes and, although kNN did a perfect job, it did so by using linear segments rather than a smooth boundary. Next, I applied statistical classification using two different approaches: Quadratic discriminant analysis (QDA) and kernel density estimation (KDE), both using a Gaussian kernel. QDA is a parametric statistical approach since it uses a limited set of parameters to perform the classification. KDE is similar except that it applies a Gaussian function to each point in the two classes and sums the result. However, it is a non-parametric statistical method because it is dependent on all the points in each class rather than just a few parameters. Finally, I apply a deep feedforward neural network (DFNN) to the problem. On our 10-point problem, the QDA approach gave a smooth boundary but miss-classified some of the points, whereas the KDE and DFNN approaches both gave smooth boundaries and were both able to perfectly classify the points in each facies. But since the DFNN approach is highly dependent on the structure of the network and is time consuming to run, the KDE approach was chosen as the optimum method to apply to the real data example.
I then move to the real data example, in which seismic facies classification with the KDE approach is used to identify facies using a seismic dataset from Permian Basin. The attributes used in this example are found using pre-stack seismic inversion and consist of Vp/Vs ratio and P-impedance. Six facies are identified on the well logs for the Wolfcamp and Spaberry formations. The most probable facies and its probability of occurrence within these formations are then projected onto the original seismic volumes.