Beyond Physics in Geophysics
By John Castagna
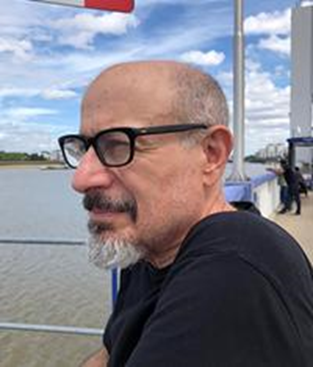
Abstract
Physics is an essential component of geophysics but there is much that physics cannot know or address. For example, physics alone cannot ascertain that an inverted low seismic impedance is indicative of a coal layer in one stratigraphic interval and the same inverted impedance represents an organic shale reservoir in another. Such geological non-uniqueness often results in the false conclusion from physics that such distinctions from seismic data are not possible. Applying physics to determine what is not possible disregards what information outside of physics can achieve. Seismology only understands relationships controlled by the wave equation and is thus incapable of predicting geological correlations between parameters and seismic attributes that are not governed by the physics of wave propagation. Generally, the more geological information that can be properly incorporated into a geophysical prediction, the better.
For the past quarter-century, explorationists have successfully empirically used seismic multi-attribute regression analysis and neural networks to make predictions that physics alone cannot achieve. More recently, deeper neural networks are being employed to perform a variety of geophysical functions. This machine learning has great promise but can also be readily misapplied and abused when used to directly make predictions, especially given the usual paucity of training data.
An alternative approach is to use machine learning to uncover relationships that may not have been foreseen because they were not readily apparent or addressed using physics alone. Once these relationships are found and understood geologically and geophysically, real, rather than artificial, intelligence can be used to predict rock and fluid properties from seismic data. For example, a simple-minded analysis of reflectivity tells us that changing the impedance contrast across an interface, or for a layer, changes the reflection amplitude of the reflected waveform but not the phase of that event. Thus, physics is readily misapplied to conclude that phase cannot be used to predict rock properties. Yet, multi-attribute analysis case studies tell us that phase is often a powerful attribute for predicting rock properties. Once artificial intelligence tells us that phase is indeed useful in this regard, it allows us to understand why the simple-minded conclusion telling us what NOT to do was wrong. Similarly, multi-attribute analysis tells us that using bandwidth extended seismic data can improve rock properties predictions even though the correlation of such data to synthetic seismograms often decreases with increasing bandwidth. Poorer correlations for bandwidth extended data have been used by those imprisoned by simple physics to conclude that bandwidth extension is not possible. Understanding when and why bandwidth extension can be useful despite such decreases in correlation falls into the realms of information theory and depositional systems in addition to geophysics. As a final example, seismic inversion theory tells us that the amplitude spectrum of the seismic wavelet must be known in order to model reflection seismic data, and thus must be known to invert that data. This apparently incontrovertible fact is very unfortunate since the temporally and spatially varying seismic wavelet spectrum is very poorly known and limits the accuracy of seismic inversion. However, using machine learning to replace the functionality of seismic inversion and inspecting the neural network weights can provide insight into what information is necessary and most robust to successfully accomplish the same task as traditional model-based seismic inversion. Such guidance can then be incorporated into a physics honoring seismic inversion scheme that works without explicit knowledge of the seismic wavelet. This is readily demonstrated with synthetic seismic data.
Bio for John Castagna
John Castagna is the Margaret S. and Robert E. Sheriff Endowed Faculty Chair in Applied Seismology at the University of Houston. He has degrees in geology, geochemistry, and geophysics and specializes in exploration geophysics. He has more than 40 years of experience in petroleum exploration including more than 20 years in academia and has published more than 100 papers in areas such as rock physics, direct hydrocarbon detection, multi-attribute analysis, and seismic inversion. Despite his ineptitude in that subject, he loves and values physics.
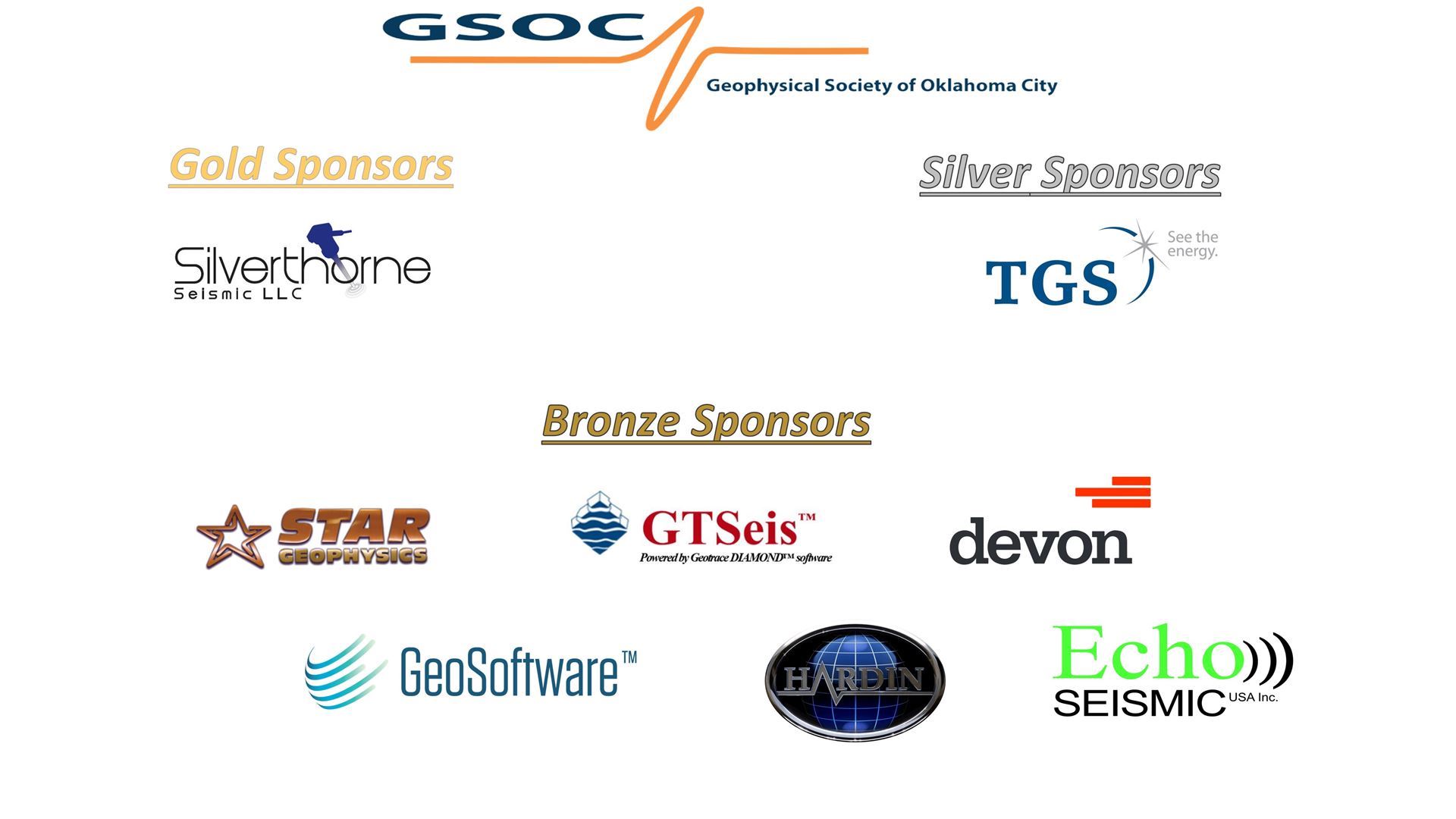